The Value of Data Governance and How to Quantify It
erwin recently hosted the second in its six-part webinar series on the practice of data governance and how to proactively deal with its complexities. Led by Frank Pörschmann of iDIGMA GmbH, an IT industry veteran and data governance strategist, watch the webinar focused on “The Value of Data Governance & How to Quantify It.”
As Mr. Pörschmann highlighted at the beginning of the series, data governance works best when it is strongly aligned with the drivers, motivations and goals of the business.
The business drivers and motivation should be the starting point for any data governance initiative. If there is no clear end goal in sight, it will be difficult to get stakeholders on board. And with many competing projects and activities vying for people’s time, it must be clear to people why choosing data governance activities will have a direct benefit to them.
“Usually we talk about benefits which are rather qualitative measures, but what we need for decision-making processes are values,” Pörschmann says. “We need quantifiable results or expected results that are fact-based. And the interesting thing with data governance, it seems to be easier for organizations and teams to state the expected benefits.”
The Data Governance Productivity Matrix
In terms of quantifying data governance, Pörschmann cites the productivity matrix as a relatively simple way to calculate real numbers. He says, “the basic assumption is if an organization equips their managers with the appropriate capabilities and instruments, then it’s management’s obligation to realize productivity potential over time.”
According to IDC, professionals who work with data spend 80 percent of their time looking for and preparing data and only 20 percent of their time on analytics.
Specifically, 80 percent of data professionals’ time is spent on data discovery, preparation and protection, and only 20 percent on analysis leading to insights.
Data governance maturity includes the ability to rely on automated and repeatable processes, which ultimately helps to increase productivity.
For example, automatically importing mappings from developers’ Excel sheets, flat files, Access and ETL tools into a comprehensive mappings inventory, complete with automatically generated and meaningful documentation of the mappings, is a powerful way to support governance while providing real insight into data movement — for data lineage and impact analysis — without interrupting system developers’ normal work methods.
When data movement has been tracked and version-controlled, it’s possible to conduct data archeology — that is, reverse-engineering code from existing XML within the ETL layer — to uncover what has happened in the past and incorporating it into a mapping manager for fast and accurate recovery.
With automation, data professionals can meet the above needs at a fraction of the cost of the traditional, manual way. To summarize, just some of the benefits of data automation are:
- Centralized and standardized code management with all automation templates stored in a governed repository
- Better quality code and minimized rework
- Business-driven data movement and transformation specifications
- Superior data movement job designs based on best practices
- Greater agility and faster time to value in data preparation, deployment and governance
- Cross-platform support of scripting languages and data movement technologies
For example, one global pharmaceutical giant reduced cost by 70 percent and generated 95 percent of production code with “zero touch.” With automation, the company improved the time to business value and significantly reduced the costly re-work associated with error-prone manual processes.
Risk Management and Regulatory Compliance
Risk management, specifically around regulatory compliance, is an important use case to demonstrate the true value of data governance.
According to Pörschmann, risk management asks two main questions.
- How likely is a specific event to happen?
- What is the impact or damage if this event happens? (e.g.m, cost of repair, cost of reputation, etc.)
“You have to understand the concept or thinking of risk officers or the risk teams,” he says. The risk teams are process-oriented, and they understand how to calculate and how to cover IT risks. But to be successful in communicating data risks with the risk management team, you need to understand how your risk teams are thinking in terms of the risk matrix.
Take the European Union’s General Data Protection Regulation (GDPR) as an example of a data cost. Your team needs to ask, “what is the likelihood that we will fail on data-based activities related to GDPR?” And then ask, “what can we do from the data side to reduce the impact or the total damage?”
But it’s not easy to design and deploy compliance in an environment that’s not well understood and difficult in which to maneuver. Data governance enables organizations to plan and document how they will discover and understand their data within context, track its physical existence and lineage, and maximize its security, quality and value.
With the right technology, organizations can automate and accelerate regulatory compliance in five steps:
- Catalog systems. Harvest, enrich/transform and catalog data from a wide array of sources to enable any stakeholder to see the interrelationships of data assets across the organization.
- Govern PII “at rest”. Classify, flag and socialize the use and governance of personally identifiable information regardless of where it is stored.
- Govern PII “in motion”. Scan, catalog and map personally identifiable information to understand how it moves inside and outside the organization and how it changes along the way.
- Manage policies and rules. Govern business terminology in addition to data policies and rules, depicting relationships to physical data catalogs and the applications that use them with lineage and impact analysis views.
- Strengthen data security. Identify regulatory risks and guide the fortification of network and encryption security standards and policies by understanding where all personally identifiable information is stored, processed and used.
It’s also important to understand that the benefits of data governance don’t stop with regulatory compliance.
A better understanding of what data you have, where it’s stored and the history of its use and access isn’t only beneficial in fending off non-compliance repercussions. In fact, such an understanding is arguably better put to use proactively.
Data governance improves data quality standards, it enables better decision-making and ensures businesses can have more confidence in the data informing those decisions.
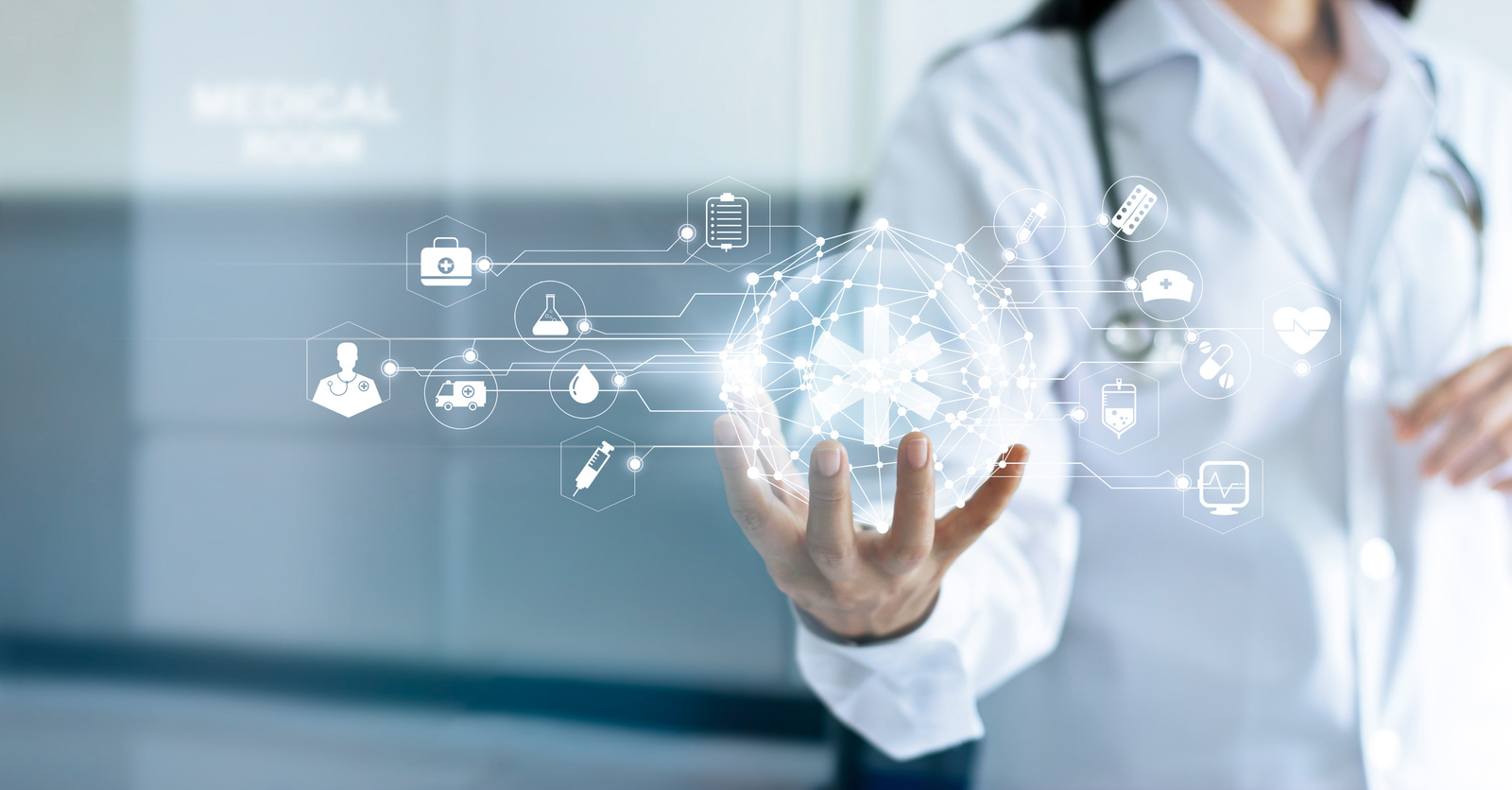