What is Data Lineage? Top 5 Benefits of Data Lineage
What is Data Lineage and Why is it Important?
Data lineage is the journey data takes from its creation through its transformations over time. It describes a certain dataset’s origin, movement, characteristics and quality.
Tracing the source of data is an arduous task.
Many large organizations, in their desire to modernize with technology, have acquired several different systems with various data entry points and transformation rules for data as it moves into and across the organization.
These tools range from enterprise service bus (ESB) products, data integration tools; extract, transform and load (ETL) tools, procedural code, application program interfaces (API)s, file transfer protocol (FTP) processes, and even business intelligence (BI) reports that further aggregate and transform data.
With all these diverse data sources, and if systems are integrated, it is difficult to understand the complicated data web they form much less get a simple visual flow. This is why data’s lineage must be tracked and why its role is so vital to business operations, providing the ability to understand where data originates, how it is transformed, and how it moves into, across and outside a given organization.
Data Lineage Use Case: From Tracing COVID-19’s Origins to Data-Driven Business
A lot of theories have emerged about the origin of the coronavirus. A recent University of California San Francisco (UCSF) study conducted a genetic analysis of COVID-19 to determine how the virus was introduced specifically to California’s Bay Area.
It detected at least eight different viral lineages in 29 patients in February and early March, suggesting no regional patient zero but rather multiple independent introductions of the pathogen. The professor who directed the study said, “it’s like sparks entering California from various sources, causing multiple wildfires.”
Much like understanding viral lineage is key to stopping this and other potential pandemics, understanding the origin of data, is key to a successful data-driven business.
Automate Data Lineage with erwin Data Intelligence
erwin was named a Leader in the Gartner 2020 Magic Quadrant for Metadata Management Solutions.
Get the report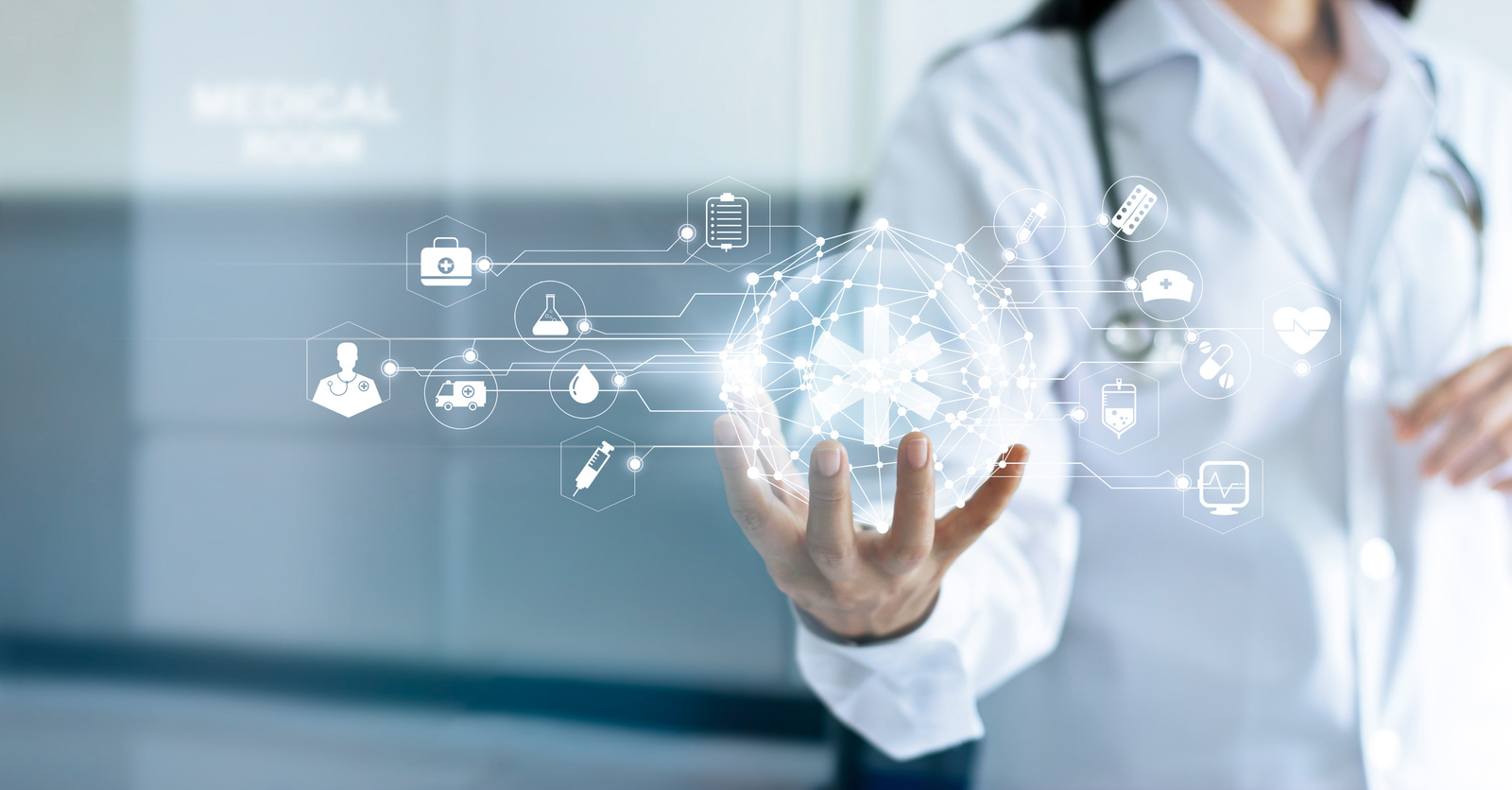
Top Five Data Lineage Benefits
From my perspective in working with customers of various sizes across multiple industries, I’d like to highlight five data lineage benefits:
1. Business Impact
Data is crucial to every organization’s survival. For that reason, businesses must think about the flow of data across multiple systems that fuel organizational decision-making.
For example, the marketing department uses demographics and customer behavior to forecast sales. The CEO also makes decisions based on performance and growth statistics. An understanding of the data’s origins and history helps answer questions about the origin of data in a Key Performance Indicator (KPI) reports, including:
- How the report tables and columns are defined in the metadata?
- Who are the data owners?
- What are the transformation rules?
Without data lineage, these functions are irrelevant, so it makes sense for a business to have a clear understanding of where data comes from, who uses it, and how it transforms. Also, when there is a change to the environment, it is valuable to assess the impacts to the enterprise application landscape.
In the event of a change in data expectations, data lineage provides a way to determine which downstream applications and processes are affected by the change and helps in planning for application updates.
2. Compliance & Auditability
Business terms and data policies should be implemented through standardized and documented business rules. Compliance with these business rules can be tracked through data lineage, incorporating auditability and validation controls across data transformations and pipelines to generate alerts when there are non-compliant data instances.
Regulatory compliance places greater transparency demands on firms when it comes to tracing and auditing data. For example, capital markets trading firms must understand their data’s origins and history to support risk management, data governance and reporting for various regulations such as BCBS 239 and MiFID II.
Also, different organizational stakeholders (customers, employees and auditors) need to be able to understand and trust reported data. Data lineage offers proof that the data provided is reflected accurately.
3. Data Governance
An automated data lineage solution stitches together metadata for understanding and validating data usage, as well as mitigating the associated risks.
It can auto-document end-to-end upstream and downstream data lineage, revealing any changes that have been made, by whom and when.
This data ownership, accountability and traceability is foundational to a sound data governance program.
See: The Benefits of Data Governance
4. Collaboration
Analytics and reporting are data-dependent, making collaboration among different business groups and/or departments crucial.
The visualization of data lineage can help business users spot the inherent connections of data flows and thus provide greater transparency and auditability.
Seeing data pipelines and information flows further supports compliance efforts.
5. Data Quality
Data quality is affected by data’s movement, transformation, interpretation and selection through people, process and technology.
Root-cause analysis is the first step in repairing data quality. Once a data steward determines where a data flaw was introduced, the reason for the error can be determined.
With data lineage and mapping, the data steward can trace the information flow backward to examine the standardizations and transformations applied to confirm whether they were performed correctly.
See Data Lineage in Action
Data lineage tools document the flow of data into and out of an organization’s systems. They capture end-to-end lineage and ensure proper impact analysis can be performed in the event of problems or changes to data assets as they move across pipelines.
The erwin Data Intelligence Suite (erwin DI) automatically generates end-to-end data lineage, down to the column level and between repositories. You can view data flows from source systems to the reporting layers, including intermediate transformation and business logic.