Data Governance and Metadata Management: You Can’t Have One Without the Other
When an organization’s data governance and metadata management programs work in harmony, then everything is easier.
Data governance is a complex but critical practice. There’s always more data to handle, much of it unstructured; more data sources, like IoT, more points of integration, and more regulatory compliance requirements.
Creating and sustaining an enterprise-wide view of and easy access to underlying metadata is also a tall order.
The numerous data types and data sources that exist today weren’t designed to work together, and data infrastructures have been cobbled together over time with disparate technologies, poor documentation and little thought for downstream integration.
Therefore, most enterprises have encountered difficulty trying to master data governance and metadata management, but they need a solid data infrastructure on which to build their applications and initiatives.
Without it, they risk faulty analyses and insights that effect not only revenue generation but regulatory compliance and any number of other organizational objectives.
Data Governance Attitudes Are Shifting
The 2020 State of Data Governance and Automation (DGA) shows that attitudes about data governance and the drivers behind it are changing – arguably for the better.
Regulatory compliance was the biggest driver for data governance implementation, according to the 2018 report. That’s not surprising given the General Data Protection Regulation (GDPR) was going into effect just six months after the survey.
Now better decision-making is the primary reason to implement data governance, cited by 60 percent of survey participants. This shift suggests organizations are using data to improve their overall performance, rather than just trying to tick off a compliance checkbox.
We’re pleased to see this because we’ve always believed that IT-siloed data governance has limited value. Instead, data governance has to be an enterprise initiative with IT and the wider business collaborating to limit data-related risks and determine where greater potential and value can be unleashed.
Metadata Management Takes Time
About 70 percent of DGA report respondents – a combination of roles from data architects to executive managers – say they spend an average of 10 or more hours per week on data-related activities.
Most of that time is spent on data analysis – but only after searching for and preparing data.
A separate study by IDC indicates data professionals actually spend 80 percent of their time on data discovery, preparation and protection and only 20 percent on analysis.
Why such a heavy lift? Finding metadata, “the data about the data,” isn’t easy.
When asked about the most significant bottlenecks in the data value chain, documenting complete data lineage leads with 62 percent followed by understanding the quality of the source data (58 percent), discovery, identification and harvesting (55 percent), and curating data assets with business context (52%.)
So it make sense that the data operations deemed most valuable in terms of automation are:
- Data Lineage (65%)
- Data Cataloging (61%)
- Data Mapping (53%)
- Impact Analysis (48%)
- Data Harvesting (38%)
- Code Generation (21%)
But as suspected, most data operations are still manual and largely dependent on technical resources. They aren’t taking advantage of repeatable, sustainable practices – also known as automation.
The Benefits of Automating Data Governance and Metadata Management Processes
Availability, quality, consistency, usability and reduced latency are requirements at the heart of successful data governance.
And with a solid framework for automation, organizations can generate metadata every time data is captured at a source, accessed by users, moved through an organization, integrated or augmented with other data from other sources, profiled, cleansed and analyzed.
Other benefits of automating data governance and metadata management processes include:
- Better Data Quality – Identification and repair of data issues and inconsistencies within integrated data sources in real time
- Quicker Project Delivery – Acceleration of Big Data deployments, Data Vaults, data warehouse modernization, cloud migration, etc.
- Faster Speed to Insights – Reversing the 80/20 rule that keeps high-paid knowledge workers too busy finding, understanding and resolving errors or inconsistencies to actually analyze source data
- Greater Productivity & Reduced Costs – Use of automated, repeatable processes to for metadata discovery, data design, data conversion, data mapping and code generation
- Digital Transformation – Better understanding of what data exists and its potential value to improve digital experiences, enhance digital operations, drive digital innovation and build digital ecosystems
- Enterprise Collaboration – The ability for IT and the wider business to find, trust and use data to effectively meet organizational objectives
Enterprise Architecture & Business Process Modeling
erwin Evolve is a single yet powerful software suite serving both EA and BP use cases.
Start Trial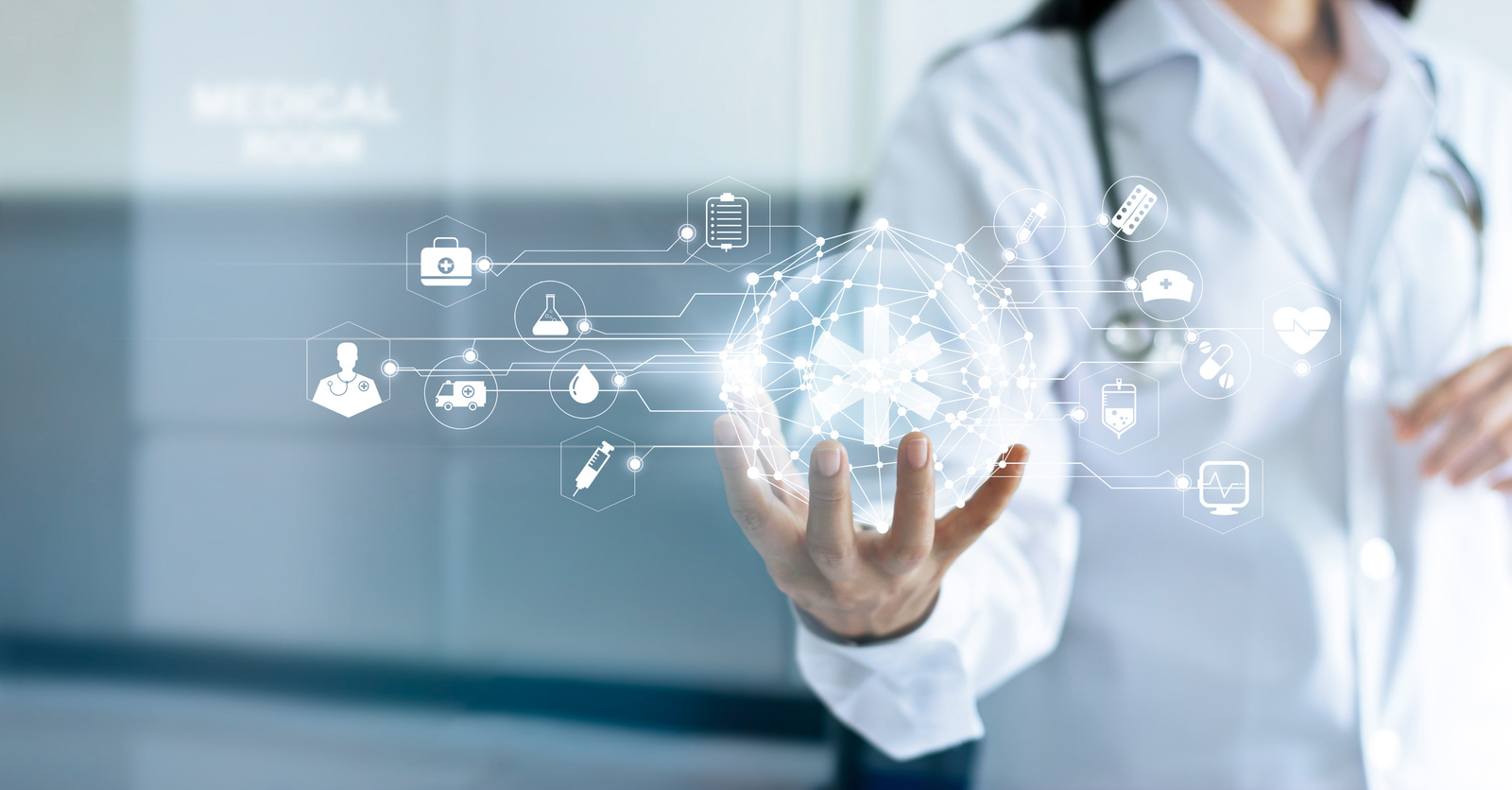