Data Intelligence and Its Role in Combating Covid-19
Data intelligence has a critical role to play in the supercomputing battle against Covid-19.
Last week, The White House announced the launch of the COVID-19 High Performance Computing Consortium, a public-private partnership to provide COVID-19 researchers worldwide with access to the world’s most powerful high performance computing resources that can significantly advance the pace of scientific discovery in the fight to stop the virus.
Rensselaer Polytechnic Institute (RPI) is one of the organizations that has joined the consortium to provide computing resources to help fight the pandemic.
While leveraging supercomputing power is a tremendous asset in our fight to combat this global pandemic, in order to deliver life-saving insights, you really have to understand what data you have and where it came from. Answering these questions is at the heart of data intelligence.
Managing and Governing Data From Lots of Disparate Sources
Collecting and managing data from many disparate sources for the Covid-19 High Performance Computing Consortium is on a scale beyond comprehension and, quite frankly, it boggles the mind to even think about it.
To feed the supercomputers with epidemiological data, the information will flow-in from many different and heavily regulated data sources, including population health, demographics, outbreak hotspots and economic impacts.
This data will be collected from organizations such as, the World Health Organization (WHO), the Centers for Disease Control (CDC), and state and local governments across the globe.
Privately it will come from hospitals, labs, pharmaceutical companies, doctors and private health insurers. It also will come from HL7 hospital data, claims administration systems, care management systems, the Medicaid Management Information System, etc.
These numerous data types and data sources most definitely weren’t designed to work together. As a result, the data may be compromised, rendering faulty analyses and insights.
To marry the epidemiological data to the population data it will require a tremendous amount of data intelligence about the:
- Source of the data;
- Currency of the data;
- Quality of the data; and
- How it can be used from an interoperability standpoint.
To do this, the consortium will need the ability to automatically scan and catalog the data sources and apply strict data governance and quality practices.
Unraveling Data Complexities with Metadata Management
Collecting and understanding this vast amount of epidemiological data in the fight against Covid-19 will require data governance oversite and data intelligence to unravel the complexities of the underlying data sources. To be successful and generate quality results, this consortium will need to adhere to strict disciplines around managing the data that comes into the study.
Metadata management will be critical to the process for cataloging data via automated scans. Essentially, metadata management is the administration of data that describes other data, with an emphasis on associations and lineage. It involves establishing policies and processes to ensure information can be integrated, accessed, shared, linked, analyzed and maintained.
While supercomputing can be used to process incredible amounts of data, a comprehensive data governance strategy plus technology will enable the consortium to determine master data sets, discover the impact of potential glossary changes, audit and score adherence to rules and data quality, discover risks, and appropriately apply security to data flows, as well as publish data to the right people.
Metadata management delivers the following capabilities, which are essential in building an automated, real-time, high-quality data pipeline:
- Reference data management for capturing and harmonizing shared reference data domains
- Data profiling for data assessment, metadata discovery and data validation
- Data quality management for data validation and assurance
- Data mapping management to capture the data flows, reconstruct data pipelines, and visualize data lineage
- Data lineage to support impact analysis
- Data pipeline automation to help develop and implement new data pipelines
- Data cataloging to capture object metadata for identified data assets
- Data discovery facilitated via a shared environment allowing data consumers to understand the use of data from a wide array of sources
Supercomputing will be very powerful in helping fight the COVID-19 virus. However, data scientists need access to quality data harvested from many disparate data sources that weren’t designed to work together to deliver critical insights and actionable intelligence.
Automated metadata harvesting, data cataloging, data mapping and data lineage combined with integrated business glossary management and self-service data discovery can give this important consortium data asset visibility and context so they have the relevant information they need to help us stop this virus effecting all of us around the globe.
To learn more about more about metadata management capabilities, download this white paper, Metadata Management: The Hero in Unleashing Enterprise Data’s Value.
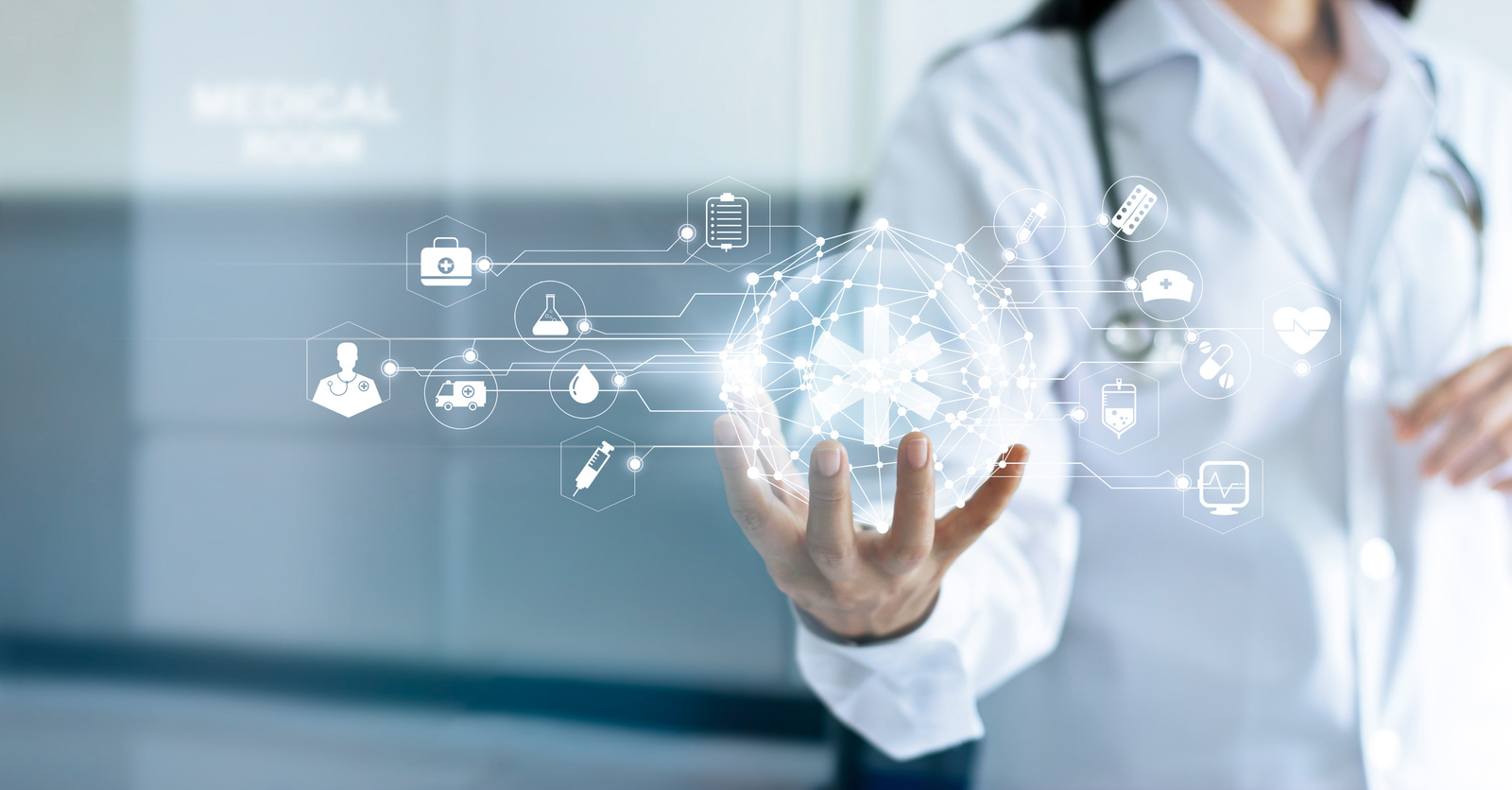