Data Preparation and Data Mapping: The Glue Between Data Management and Data Governance to Accelerate Insights and Reduce Risks
Organizations have spent a lot of time and money trying to harmonize data across diverse platforms, including cleansing, uploading metadata, converting code, defining business glossaries, tracking data transformations and so on. But the attempts to standardize data across the entire enterprise haven’t produced the desired results.
A company can’t effectively implement data governance – documenting and applying business rules and processes, analyzing the impact of changes and conducting audits – if it fails at data management.
The problem usually starts by relying on manual integration methods for data preparation and mapping. It’s only when companies take their first stab at manually cataloging and documenting operational systems, processes and the associated data, both at rest and in motion, that they realize how time-consuming the entire data prepping and mapping effort is, and why that work is sure to be compounded by human error and data quality issues.
To effectively promote business transformation, as well as fulfil regulatory and compliance mandates, there can’t be any mishaps.
It’s obvious that the manual road is very challenging to discover and synthesize data that resides in different formats in thousands of unharvested, undocumented databases, applications, ETL processes and procedural code.
Consider the problematic issue of manually mapping source system fields (typically source files or database tables) to target system fields (such as different tables in target data warehouses or data marts).
These source mappings generally are documented across a slew of unwieldy spreadsheets in their “pre-ETL” stage as the input for ETL development and testing. However, the ETL design process often suffers as it evolves because spreadsheet mapping data isn’t updated or may be incorrectly updated thanks to human error. So questions linger about whether transformed data can be trusted.
Data Quality Obstacles
The sad truth is that high-paid knowledge workers like data scientists spend up to 80 percent of their time finding and understanding source data and resolving errors or inconsistencies, rather than analyzing it for real value.
Statistics are similar when looking at major data integration projects, such as data warehousing and master data management with data stewards challenged to identify and document data lineage and sensitive data elements.
So how can businesses produce value from their data when errors are introduced through manual integration processes? How can enterprise stakeholders gain accurate and actionable insights when data can’t be easily and correctly translated into business-friendly terms?
How can organizations master seamless data discovery, movement, transformation and IT and business collaboration to reverse the ratio of preparation to value delivered.
What’s needed to overcome these obstacles is establishing an automated, real-time, high-quality and metadata- driven pipeline useful for everyone, from data scientists to enterprise architects to business analysts to C-level execs.
Doing so will require a hearty data management strategy and technology for automating the timely delivery of quality data that measures up to business demands.
From there, they need a sturdy data governance strategy and technology to automatically link and sync well-managed data with core capabilities for auditing, statutory reporting and compliance requirements as well as to drive business insights.
Creating a High-Quality Data Pipeline
Working hand-in-hand, data management and data governance provide a real-time, accurate picture of the data landscape, including “data at rest” in databases, data lakes and data warehouses and “data in motion” as it is integrated with and used by key applications. And there’s control of that landscape to facilitate insight and collaboration and limit risk.
With a metadata-driven, automated, real-time, high-quality data pipeline, all stakeholders can access data that they now are able to understand and trust and which they are authorized to use. At last they can base strategic decisions on what is a full inventory of reliable information.
The integration of data management and governance also supports industry needs to fulfill regulatory and compliance mandates, ensuring that audits are not compromised by the inability to discover key data or by failing to tag sensitive data as part of integration processes.
Data-driven insights, agile innovation, business transformation and regulatory compliance are the fruits of data preparation/mapping and enterprise modeling (business process, enterprise architecture and data modeling) that revolves around a data governance hub.
erwin Data Catalog combines data management and data governance processes in an automated flow through the integration lifecycle from data mapping for harmonization and aggregation to generating the physical embodiment of data lineage – that is the creation, movement and transformation of transactional and operational data.
Its hallmark is a consistent approach to data delivery (business glossaries connect physical metadata to specific business terms and definitions) and metadata management (via data mappings).
How to automate data mapping
Easily automate data mapping and code generation with erwin Data Catalog
Request Demo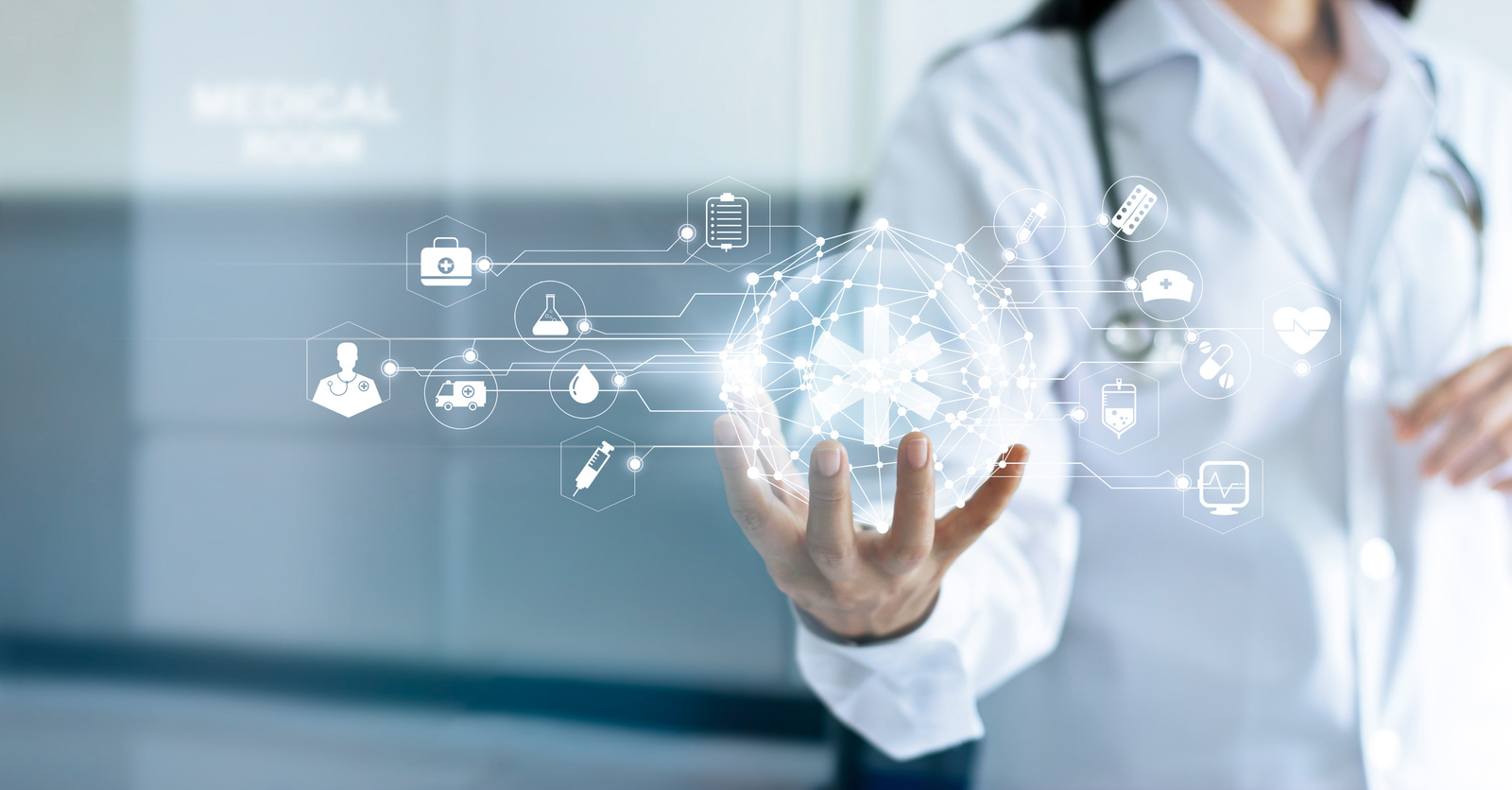