How Metadata Makes Data Meaningful
Metadata is an important part of data governance, and as a result, most nascent data governance programs are rife with project plans for assessing and documenting metadata. But in many scenarios, it seems that the underlying driver of metadata collection projects is that it’s just something you do for data governance.
So most early-stage data governance managers kick off a series of projects to profile data, make inferences about data element structure and format, and store the presumptive metadata in some metadata repository. But are these rampant and often uncontrolled projects to collect metadata properly motivated?
There is rarely a clear directive about how metadata is used. Therefore prior to launching metadata collection tasks, it is important to specifically direct how the knowledge embedded within the corporate metadata should be used.
Managing metadata should not be a sub-goal of data governance. Today, metadata is the heart of enterprise data management and governance/ intelligence efforts and should have a clear strategy – rather than just something you do.
What Is Metadata?
Quite simply, metadata is data about data. It’s generated every time data is captured at a source, accessed by users, moved through an organization, integrated or augmented with other data from other sources, profiled, cleansed and analyzed. Metadata is valuable because it provides information about the attributes of data elements that can be used to guide strategic and operational decision-making. It answers these important questions:
- What data do we have?
- Where did it come from?
- Where is it now?
- How has it changed since it was originally created or captured?
- Who is authorized to use it and how?
- Is it sensitive or are there any risks associated with it?
The Role of Metadata in Data Governance
Organizations don’t know what they don’t know, and this problem is only getting worse. As data continues to proliferate, so does the need for data and analytics initiatives to make sense of it all. Here are some benefits of metadata management for data governance use cases:
- Better Data Quality: Data issues and inconsistencies within integrated data sources or targets are identified in real time to improve overall data quality by increasing time to insights and/or repair.
- Quicker Project Delivery: Accelerate Big Data deployments, Data Vaults, data warehouse modernization, cloud migration, etc., by up to 70 percent.
- Faster Speed to Insights: Reverse the current 80/20 rule that keeps high-paid knowledge workers too busy finding, understanding and resolving errors or inconsistencies to actually analyze source data.
- Greater Productivity & Reduced Costs: Being able to rely on automated and repeatable metadata management processes results in greater productivity. Some erwin customers report productivity gains of 85+% for coding, 70+% for metadata discovery, up to 50% for data design, up to 70% for data conversion, and up to 80% for data mapping.
- Regulatory Compliance: Regulations such as GDPR, HIPAA, PII, BCBS and CCPA have data privacy and security mandates, so sensitive data needs to be tagged, its lineage documented, and its flows depicted for traceability.
- Digital Transformation: Knowing what data exists and its value potential promotes digital transformation by improving digital experiences, enhancing digital operations, driving digital innovation and building digital ecosystems.
- Enterprise Collaboration: With the business driving alignment between data governance and strategic enterprise goals and IT handling the technical mechanics of data management, the door opens to finding, trusting and using data to effectively meet organizational objectives.
Giving Metadata Meaning
So how do you give metadata meaning? While this sounds like a deep philosophical question, the reality is the right tools can make all the difference.
erwin Data Intelligence (erwin DI) combines data management and data governance processes in an automated flow.
It’s unique in its ability to automatically harvest, transform and feed metadata from a wide array of data sources, operational processes, business applications and data models into a central data catalog and then make it accessible and understandable within the context of role-based views.
erwin DI sits on a common metamodel that is open, extensible and comes with a full set of APIs. A comprehensive list of erwin-owned standard data connectors are included for automated harvesting, refreshing and version-controlled metadata management. Optional erwin Smart Data Connectors reverse-engineer ETL code of all types and connect bi-directionally with reporting and other ecosystem tools. These connectors offer the fastest and most accurate path to data lineage, impact analysis and other detailed graphical relationships.
Additionally, erwin DI is part of the larger erwin EDGE platform that integrates data modeling, enterprise architecture, business process modeling, data cataloging and data literacy. We know our customers need an active metadata-driven approach to:
- Understand their business, technology and data architectures and the relationships between them
- Create an automate a curated enterprise data catalog, complete with physical assets, data models, data movement, data quality and on-demand lineage
- Activate their metadata to drive agile and well-governed data preparation with integrated business glossaries and data dictionaries that provide business context for stakeholder data literacy
erwin was named a Leader in Gartner’s “2019 Magic Quadrant for Metadata Management Solutions.”
Click here to get a free copy of the report.
Click here to request a demo of erwin DI.
Gartner's Magic Quadrant for Metadata Management Solutions
Get the Report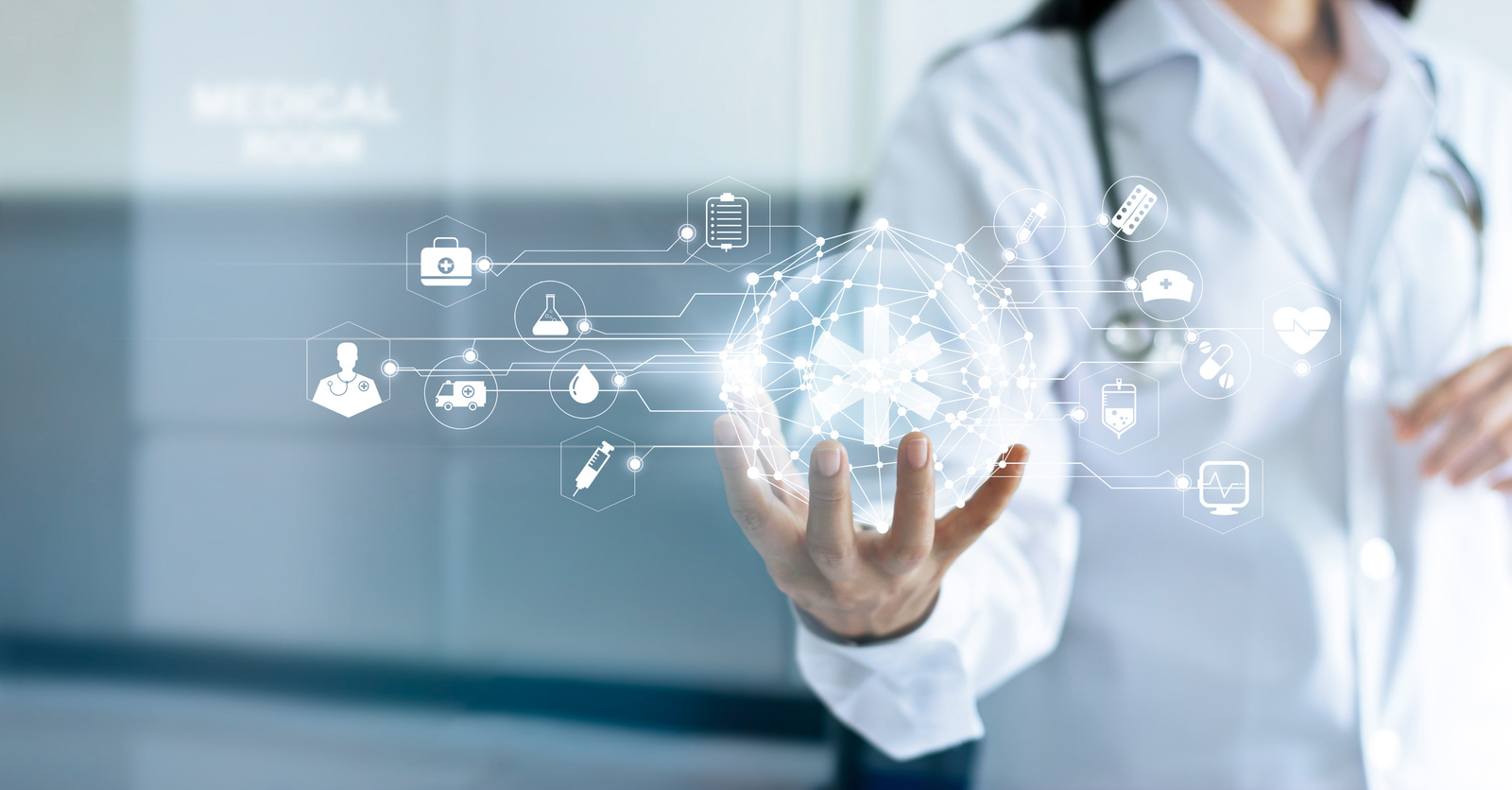