5 Ways Data Modeling Is Critical to Data Governance
Enterprises are trying to manage data chaos. They might have 300 applications, with 50 different databases and a different schema for each one.
They also face increasing regulatory pressure because of global data regulations, such as the European Union’s General Data Protection Regulation (GDPR) and the new California Consumer Privacy Act (CCPA), that went into effect last week on Jan. 1.
Then there’s unstructured data with no contextual framework to govern data flows across the enterprise not to mention time-consuming manual data preparation and limited views of data lineage.
For decades, data modeling has been the optimal way to design and deploy new relational databases with high-quality data sources and support application development. It is a tried-and-true practice for lowering data management costs, reducing data-related risks, and improving the quality and agility of an organization’s overall data capability.
And the good news is that it just keeps getting better. Today’s data modeling is not your father’s data modeling software.
While it’s always been the best way to understand complex data sources and automate design standards and integrity rules, the role of data modeling continues to expand as the fulcrum of collaboration between data generators, stewards and consumers.
That’s because it’s the best way to visualize metadata, and metadata is now the heart of enterprise data management and data governance/ intelligence efforts.
So here’s why data modeling is so critical to data governance.
1. Uncovering the connections between disparate data elements: Visualize metadata and schema to mitigate complexity and increase data literacy and collaboration across a broad range of data stakeholders. Because data modeling reduces complexity, all members of the team can work around a data model to better understand and contribute to the project.
2. Capturing and sharing how the business describes and uses data: Create and integrate business and semantic metadata to augment and accelerate data intelligence and governance efforts. Data modeling captures how the business uses data and provides context to the data source.
3. Deploying higher quality data sources with the appropriate structural veracity: Automate and enforce data model design tasks to ensure data integrity. From regulatory compliance and business intelligence to target marketing, data modeling maintains an automated connection back to the source.
4. Building a more agile and governable data architecture: Create and implement common data design standards from the start. Data modeling standardizes design tasks to improve business alignment and simplify integration.
5. Governing the design and deployment of data across the enterprise: Manage the design and maintenance lifecycle for data sources. Data modeling provides visibility, management and full version control over the lifecycle for data design, definition and deployment.
erwin Data Modeler: Where the Magic Happens
erwin has just released a new version of erwin DM, the world’s No. 1 data modeling software for designing, deploying and understanding data sources to meet modern business needs. erwin DM 2020 is an essential source of metadata and a critical enabler of data governance and intelligence efforts.
The new version of erwin DM includes these features:
- A modern, configurable workspace so users can customize the modeling canvas and optimize access to features and functionality that best support their workflows
- Support for and model integration from major databases to work effectively across platforms and reuse work product, including native support for Amazon Redshift and updated support for the latest DB2 releases and certification for the latest MS SQL Server releases
- Model exchange (import/export) to/from a wide variety of data management environments
- Modeling task automation that saves modelers time, reduces errors and increases work product quality and speed, including a new scheduler to automate the offline reverse-engineering of databases into data models
- New Quick Compare templates as part of the Complete Compare feature to compare and synchronize data models and sources
- New ODBC query tool for creating and running custom model and metadata reports
- Design transformations to customize and automate super-type/sub-type relationships between logical and physical models
erwin DM also integrates with the erwin Data Intelligence Suite (erwin DI) to automatically harvest the metadata in erwin data models for ingestion into the data catalog for better analytics, governance and overall data intelligence.
The role of data modeling in the modern data-driven business continues to expand with the benefits long-realized by database professionals and developers now experienced by a wider range of architects, business analysts and data administrators in a variety of data-centric initiatives.
Click here to test drive of the new erwin DM.
erwin Data Modeler has new features!
A modern and customizable workspace, model integration support (eg Amazon Redshift) and modeling task automation.
Try It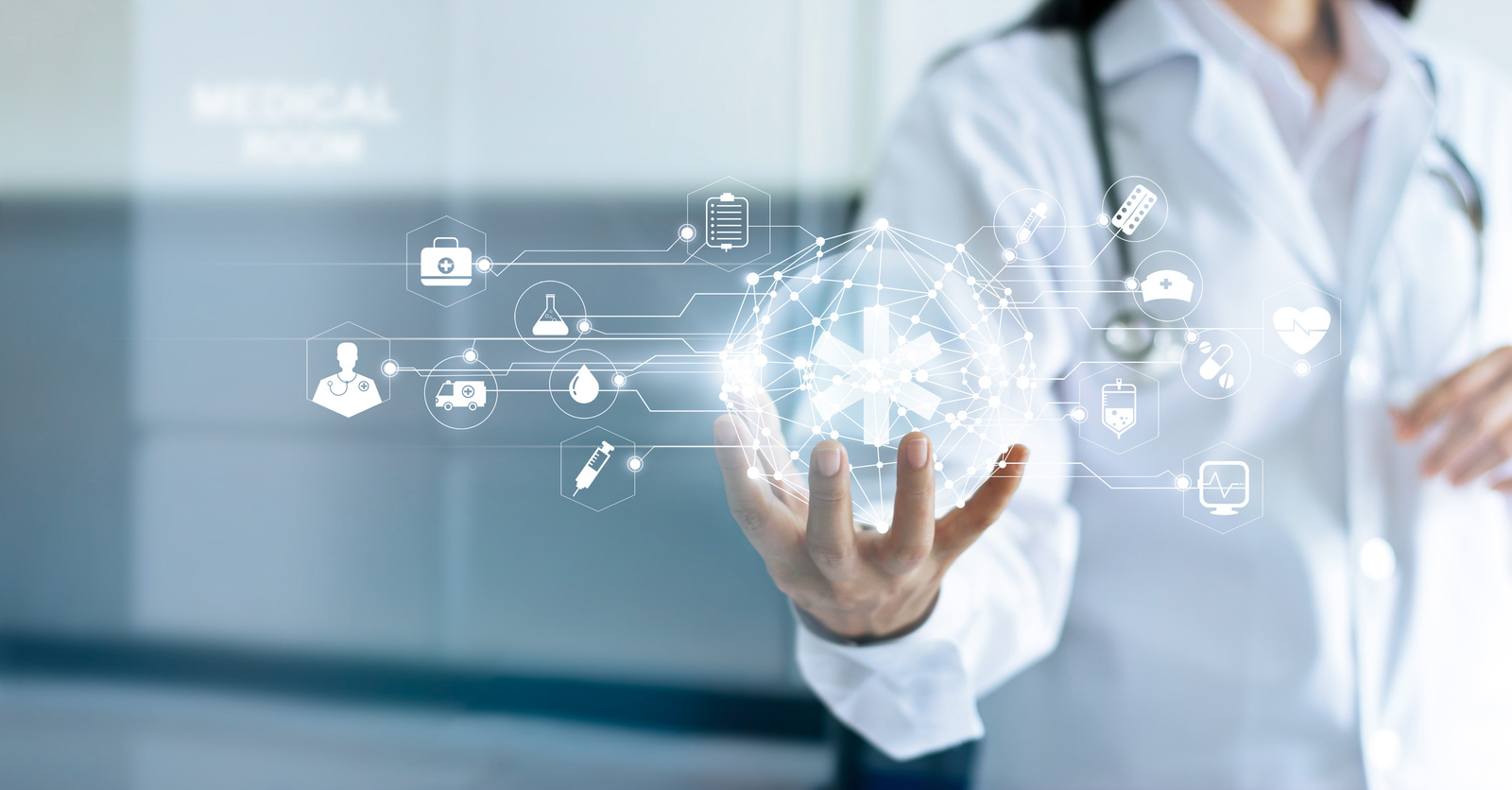