Data Governance Stock Check: Using Data Governance to Take Stock of Your Data Assets
For regulatory compliance (e.g., GDPR) and to ensure peak business performance, organizations often bring consultants on board to help take stock of their data assets. This sort of data governance “stock check” is important but can be arduous without the right approach and technology. That’s where data governance comes in …
While most companies hold the lion’s share of operational data within relational databases, it also can live in many other places and various other formats. Therefore, organizations need the ability to manage any data from anywhere, what we call our “any-squared” (Any2) approach to data governance.
Any2 first requires an understanding of the ‘3Vs’ of data – volume, variety and velocity – especially in context of the data lifecycle, as well as knowing how to leverage the key capabilities of data governance – data cataloging, data literacy, business process, enterprise architecture and data modeling – that enable data to be leveraged at different stages for optimum security, quality and value.
Following are two examples that illustrate the data governance stock check, including the Any2 approach in action, based on real consulting engagements.
Data Governance “Stock Check” Case 1: The Data Broker
This client trades in information. Therefore, the organization needed to catalog the data it acquires from suppliers, ensure its quality, classify it, and then sell it to customers. The company wanted to assemble the data in a data warehouse and then provide controlled access to it.
The first step in helping this client involved taking stock of its existing data. We set up a portal so data assets could be registered via a form with basic questions, and then a central team received the registrations, reviewed and prioritized them. Entitlement attributes also were set up to identify and profile high-priority assets.
A number of best practices and technology solutions were used to establish the data required for managing the registration and classification of data feeds:
1. The underlying metadata is harvested followed by an initial quality check. Then the metadata is classified against a semantic model held in a business glossary.
2. After this classification, a second data quality check is performed based on the best-practice rules associated with the semantic model.
3. Profiled assets are loaded into a historical data store within the warehouse, with data governance tools generating its structure and data movement operations for data loading.
4. We developed a change management program to make all staff aware of the information brokerage portal and the importance of using it. It uses a catalog of data assets, all classified against a semantic model with data quality metrics to easily understand where data assets are located within the data warehouse.
5. Adopting this portal, where data is registered and classified against an ontology, enables the client’s customers to shop for data by asset or by meaning (e.g., “what data do you have on X topic?”) and then drill down through the taxonomy or across an ontology. Next, they raise a request to purchase the desired data.
This consulting engagement and technology implementation increased data accessibility and capitalization. Information is registered within a central portal through an approved workflow, and then customers shop for data either from a list of physical assets or by information content, with purchase requests also going through an approval workflow. This, among other safeguards, ensures data quality.
The benefits of Data Governance
How an enterprise data governance experience approach to data governance can be your organization edge.
Request Demo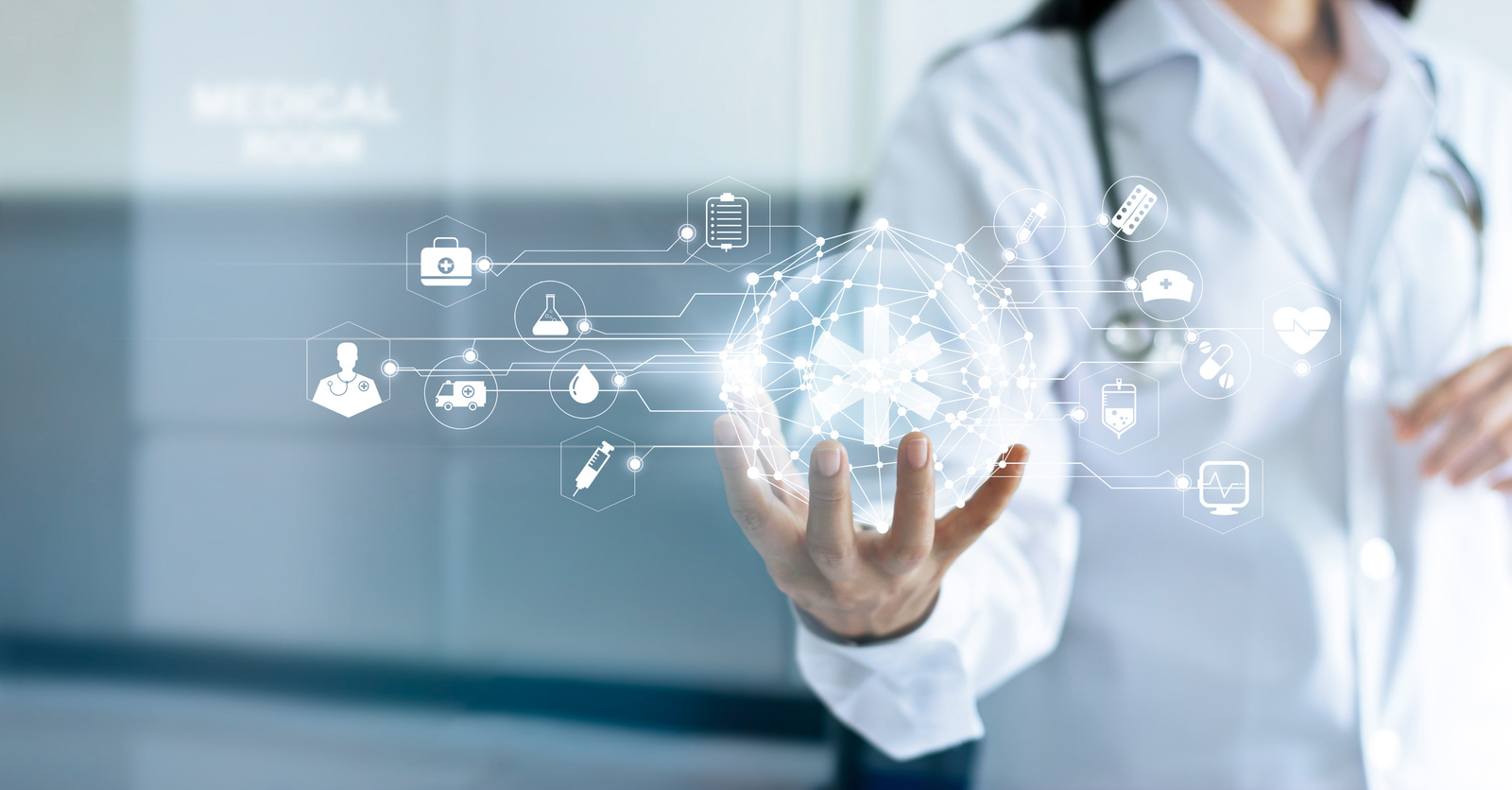
Data Governance “Stock Check” Case 2: Tracking Rogue Data
This client has a geographically-dispersed organization that stored many of its key processes in Microsoft Excel TM spreadsheets. They were planning to move to Office 365TM and were concerned about regulatory compliance, including GDPR mandates.
Knowing that electronic documents are heavily used in key business processes and distributed across the organization, this company needed to replace risky manual processes with centralized, automated systems.
A key part of the consulting engagement was to understand what data assets were in circulation and how they were used by the organization. Then process chains could be prioritized to automate and outline specifications for the system to replace them.
This organization also adopted a central portal that allowed employees to register data assets. The associated change management program raised awareness of data governance across the organization and the importance of data registration.
For each asset, information was captured and reviewed as part of a workflow. Prioritized assets were then chosen for profiling, enabling metadata to be reverse-engineered before being classified against the business glossary.
Additionally, assets that were part of a process chain were gathered and modeled with enterprise architecture (EA) and business process (BP) modeling tools for impact analysis.
High-level requirements for new systems then could be defined again in the EA/BP tools and prioritized on a project list. For the others, decisions could be made on whether they could safely be placed in the cloud and whether macros would be required.
In this case, the adoption of purpose-built data governance solutions helped build an understanding of the data assets in play, including information about their usage and content to aid in decision-making.
This client then had a good handle of the “what” and “where” in terms of sensitive data stored in their systems. They also better understood how this sensitive data was being used and by whom, helping reduce regulatory risks like those associated with GDPR.
In both scenarios, we cataloged data assets and mapped them to a business glossary. It acts as a classification scheme to help govern data and located data, making it both more accessible and valuable. This governance framework reduces risk and protects its most valuable or sensitive data assets.
Focused on producing meaningful business outcomes, the erwin EDGE platform was pivotal in achieving these two clients’ data governance goals – including the infrastructure to undertake a data governance stock check. They used it to create an “enterprise data governance experience” not just for cataloging data and other foundational tasks, but also for a competitive “EDGE” in maximizing the value of their data while reducing data-related risks.